Artificial intelligence has been the driving force behind a lot of huge developments in the last year. But while super-intelligent chatbots and rapid art generation have gripped the internet, AI has gone as far as to fight back against one of humanity's biggest problems: ageing.
Through a recent development from researchers at the University of Edinburgh, machine-learning systems have been used in the world of drug discovery, unearthing a selection of new anti-ageing medicines.
A branch of AI, machine learning focuses on using data to imitate the way that humans learn, building on accuracy the more data it is fed. In the past, it’s been used to create chess-playing robots, self-driving cars and even on-demand TV recommendations, but this particular algorithm was looking for a new senolytics medicine.
Senolytics are, essentially, a form of drug that can slow ageing, as well as preventing age-related diseases. They work by killing off cells known as senescent cells – damaged cells that, while unable to multiply, can release substances that cause inflammation.
While powerful medicines, senolytics can be expensive – and time-intensive – to develop. Noticing this, Vanessa Smer-Barreto, a research fellow for the Institute of Genetics and Molecular Medicine at the University of Edinburgh, turned to machine learning.
“Generating your own biological data can be really expensive, and it can take up a lot of time, even just to gather training data,” she explains.
“What made our approach different to others is that we tried to do it on limited funds. We took training data from existing literature and looked into how to utilise this with machine learning to speed things up.”
By using a machine learning algorithm, she was able to find three promising options for these types of drugs.
To do this, Smer-Barreto (along with her colleagues) fed an AI model examples of known senolytics and non-senolytics, teaching the model to distinguish between the two. This could then be used to predict whether molecules they hadn’t seen before could be senolytics based on if they matched up with the pre-fed examples.
Around 80 senolytics are known, but of that number, just two have been tested in humans. While that sounds like a tiny percentage, it takes 10 to 20 years for drugs to reach the market, along with huge funds.
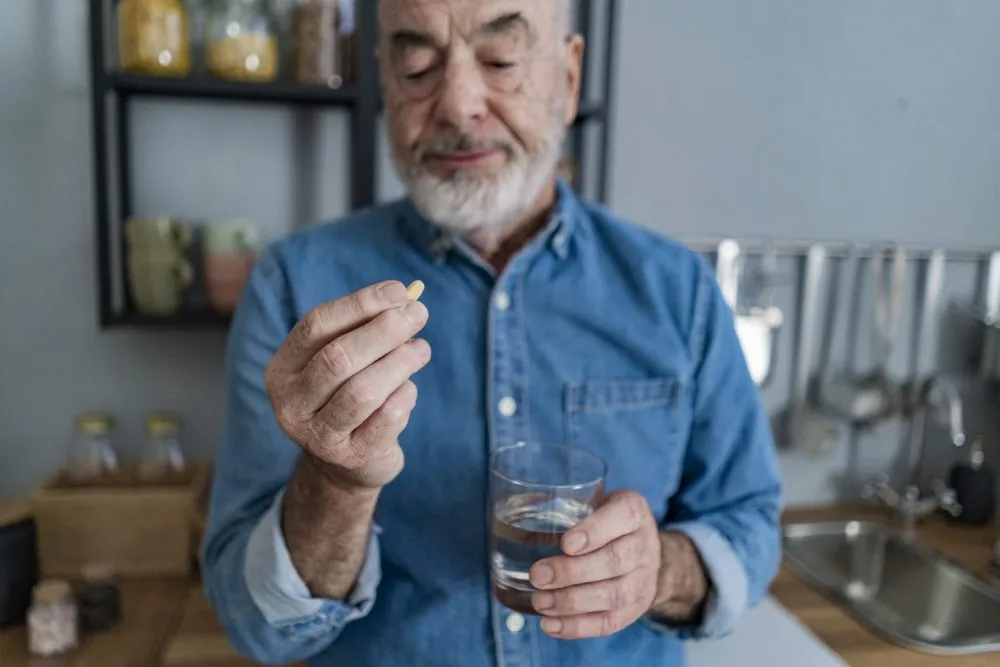
The team read through a wide range of papers but were selective with the results, limiting themselves to just 58 compounds. By doing this, they cut out any compounds where the results weren’t perfectly clear.
A total of 4,340 molecules were fed into the machine-learning model, returning a list of results in just five minutes. The model had identified 21 top-scoring molecules which it deemed likely to be senolytics. Without the machine-learning model, this process could take weeks and huge sums of money to get these results.
Finally, the potential drug candidates were tested on two types of cells: healthy and ageing.
Of the 21 top-scoring molecules, there were three able to eliminate the ageing cells, while still keeping normal cells alive. These new senolytics were then put under further testing to understand more about the way they interact with the body.
While the study was successful, it is just the start for this research. “The next step is to team up with clinicians at my university to try testing the drugs we discovered on their samples of robust human lung tissue,” explains Smer-Baretto.
Through these future tests, the team hopes to see whether they can fight ageing on the tissue of damaged organs. Smer-Baretto points out that the patient isn’t necessarily going to be given a big dose of a drug, especially in earlier stages. These drugs are also being tested on tissue models first, and drugs could be administered locally or micro-dosed.
“It is essential that with any drug that we are administering or experimenting with, we consider the fact that it may do more harm than good,” says Smer-Baretto.
“The drugs have to go through many stages first, and even if they make it through to the market, it will have gone through a host of safety concerns tests first.”
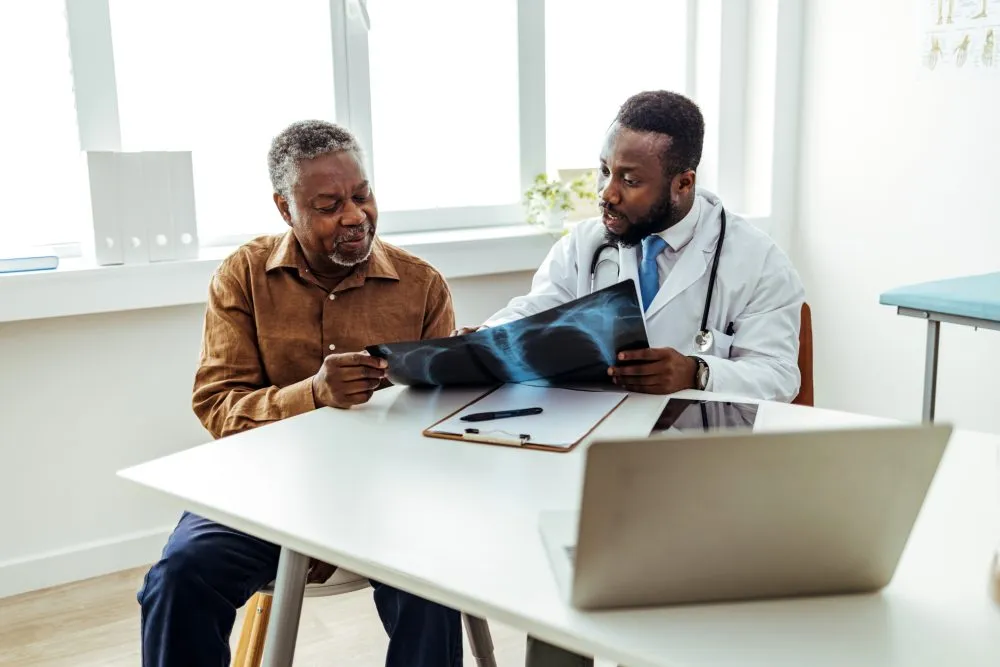
While this method of examining data was put to work on drugs related to ageing, there is nothing stopping the AI from being deployed in other areas.
“We had a very specific approach with the data, but there is nothing stopping us from applying similar techniques towards other diseases such as cancer. We’re keen to explore all avenues."
Read more: