Linnaeus’s system of binomial classification is the one that scientists still use today to classify humans and all other living things. But Linnaeus’s system didn’t just include the category of Homo sapiens, as it turns out.
It also incorrectly — but as historians would tell you, unsurprisingly — included five subcategories of humans separated by race. (One of these five was set aside for mythological humans who didn’t exist in real life, in case you’re still ready to get behind his science.)
But Linnaeus’s classification system wasn’t even the worst of the lot. Over the course of the 18th Century, increasingly racist systems of classification began to emerge, along with pseudosciences like eugenics and physiognomy.
These allowed elite white men to provide a “scientific” basis for the differential treatment of people of colour, women, disabled people, and gay people, among other groups. Although those fields have long since been discredited, their legacy is still visible in instances as far-ranging as the maternal health outcomes to the divergent rates of car insurance that are offered to black vs. white drivers, as described in an investigation conducted by ProPublica and Consumer Reports.
Read more about algorithms:
What’s more, as machine learning techniques are increasingly extended into new domains of human life, scientific racism is itself returning. Pointing to and debunking one machine learning technique that employs images of faces in an attempt to classify criminals, three prominent artificial intelligence researchers — Blaise Agüera y Arcas, Margaret Mitchell, and Alexander Todorov — have asserted that scientific racism has “entered a new era.”
A simple solution might be to say, “Fine, then. Let’s just not classify anything or anyone!” But the flaw in that plan is that data must be classified in some way to be put to use.
In fact, by the time that information becomes data, it’s already been classified in some way. Data, after all, is information made tractable, to borrow a term from computer science. “What distinguishes data from other forms of information is that it can be processed by a computer, or by computer-like operations,” as Lauren has written in an essay co-authored with information studies scholar Miriam Posner.
And to enable those operations, which range from counting to sorting and from modelling to visualising, the data must be placed into some kind of category — if not always into a conceptual category like gender, then at the least into a computational category like Boolean (a type of data with only two values, like true or false), integer (a type of number with no decimal points, like 237 or −1), or string (a sequence of letters or words, like “this”).
Classification systems are essential to any working infrastructure, as information theorists Geoffrey Bowker and Susan Leigh Star have argued in their influential book Sorting Things Out. This is true not only for computational infrastructures and conceptual ones, but also for physical infrastructures like the checkout line at the grocery store.
Think about how angry a shopper can get when they’re stuck in the express line behind someone with more than the designated 15 items or less. Or, closer to home, think of the system you use (or should use) to sort your clothes for the wash.
It’s not that we should reject these classification systems out of hand, or even that we could if we wanted to. (We’re pretty sure that no one wants all their socks to turn pink.) It’s just that once a system is in place, it becomes naturalised as “the way things are.”
This means we don’t question how our classification systems are constructed, what values or judgments might be encoded into them, or why they were thought up in the first place. In fact—and this is another point made by Bowker and Star—we often forget to ask these questions until our systems become objects of contention, or completely break down.
Read more about scientific racism:
- Race science: Angela Saini on the creeping resurgence of racism in science
- Disability rights activist Adam Pearson on the lasting effect of eugenics
Bowker and Star give the example of the public debates that took place in the 1990s around the categories of race employed on the US Federal Census. At issue was whether people should be able to choose multiple races on the census form.
Multiracial people and their families were some of the main proponents of the option, who saw it as a way to recognise their multiple identities rather than forcing them to squeeze themselves into a single, inadequate box. Those opposed included the Congressional Black Caucus as well as some Black and Latinx civil rights groups that saw the option as potentially reducing their representative voice.
Ultimately, the 2000 census did allow people to choose multiple races, and millions of people took advantage of it. But the debates around that single category illustrate how classification gets complicated quickly, and with a range of personal and political stakes.
Classification systems also carry significant material consequences, and the US Census provides an additional example of that. Census counts are used to draw voting districts, make policy decisions, and allocate billions of dollars in federal resources.
The recent Republican-led proposal to introduce a question about citizenship status on the 2020 census represents an attempt to wield this power to very pointed political ends. Because undocumented immigrants know the risks, like deportation, that come with being counted, they are less likely to complete the census questionnaire.
But because both political representation and federal funding are allocated according to the number and geographic areas of people counted in the census, undercounting undocumented immigrants (and the documented immigrants they often live with) means less voting power — and fewer resources — accorded to those groups.
This is a clear example of what we term the paradox of exposure: the double bind that places those who stand to significantly gain from being counted in the most danger from that same counting (or classifying) act.
In each of these cases, as is true of any case of not fitting (or not wanting to fit) neatly into a box, it’s important to ask whether it’s the categories that are inadequate, or whether — and this is a key feminist move — it’s the system of classification itself.
Lurking under the surface of so many classification systems are false binaries and implied hierarchies, such as the artificial distinctions between men and women, reason and emotion, nature and culture, and body and world. Decades of feminist thinking have taught us to question why these distinctions have come about; what social, cultural, or political values they reflect; what hidden (or not so hidden) hierarchies they encode; and, crucially, whether they should exist in the first place.
Questioning Classification Systems
Let’s spend some time with an actual person who has started to question the classification systems that surround him: one Michael Hicks, an eight-year-old Cub Scout from New Jersey. Why is Mikey, as he’s more commonly known, so concerned about classification?
Well, Mikey shares his name with someone who has been placed on a terrorist watch list by the US federal government. As a result, Mikey has also been classified as a potential terrorist and is subjected to the highest level of airport security screening every time that he travels.
Read more about ethics:
- Forbidden medicine: what do we do when medical breakthroughs are unethical?
- Human overpopulation: can having fewer children really make a difference?
“A terrorist can blow his underwear up and they don’t catch him. But my eight-year-old can’t walk through security without being frisked,” his mother lamented to Lizette Alvarez, a reporter for the New York Times who covered the issue in 2010.
Of course, in some ways, Mikey is lucky. He is white, so he does not run the risk of racial profiling — unlike, for example, the many Black women who receive TSA pat-downs when they wear their hair naturally.
Moreover, Mikey’s name sounds Anglo-European, so he does not need to worry about religious or ethnic profiling either — unlike, for another example, people named Muhammad who are pulled over by the police due to their Muslim name.
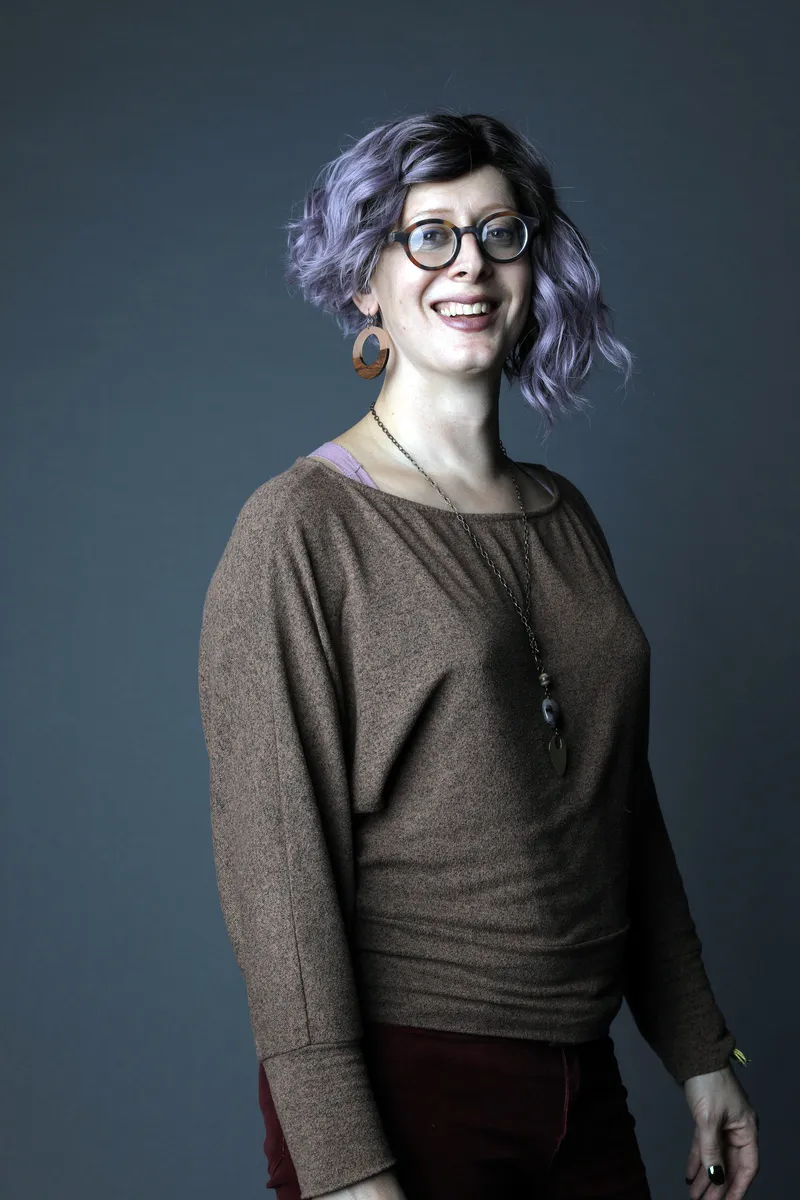
But Mikey the Cub Scout still helps to expose the brokenness of some of the categories that structure the TSA’s terrorist classification system; the combination of first and last name is simply insufficient to classify someone as a terrorist or not.
Or, consider another person with a history of bad experiences at the (literal) hands of the TSA. Sasha Costanza-Chock is nonbinary. They are also a design professor at MIT, so they have a lot of experience both living with and thinking through oppressive classification systems.
In a 2018 essay, “Design Justice, A.I., and Escape from the Matrix of Domination,” they give a concrete example of why design justice is needed in relation to data. The essay describes how the seemingly simple system employed by the operators of those hands-in-the-air millimetre-wave airport security scanning machines is in fact quite complex — and also fundamentally flawed.
Few cisgender people are aware of the fact that before you step into a scanning machine, the TSA agent operating the machine looks you up and down, decides whether you are male or female, and then pushes a button to select the corresponding gender on the scanner’s touchscreen interface.
That human decision loads the algorithmic profile for either male bodies or female ones, against which your body’s measurements are compared. If your measurements diverge from the statistical norm of that gender’s body — whether the discrepancy is because you’re concealing a deadly weapon, because your body doesn’t fit neatly into either of the two categories that the system has provided, or because the TSA agent simply made the wrong choice — you trigger a “risk alert.”
Then, in an act of what legal theorist Dean Spade terms 'administrative violence', you are subjected to the same full-body pat-down as a potential terrorist. Here it’s not that the scanning machines rely upon an insufficient number of categories, as in the case of Mikey the Cub Scout, or that they employ the wrong ones, as Mikey’s mom would likely say.

It’s that the TSA scanners shouldn’t rely on gender to classify air travellers to begin with. (And while we’re going down that path, how about we not have a state agency that systematically pathologises Black women or trans people or Cub Scouts in the first place?)
So when we say that what gets counted counts, it’s folks like Sasha Costanza-Chock or Mikey Hicks that we’re thinking about. Because flawed classification systems — like the one that underlies the airport scanner’s risk-detection algorithm or the one that determines which names end up on terrorist watch lists or simply (simply!) the gender binary — are not only significant problems in themselves, but also symptoms of a more global condition of inequality.
Data Feminism by Catherine D'Ignazio and Lauren F. Klein is out on 31 March (MIT Press, £25).